Why ELT is the Future of Data Integration
An effective method of transferring and processing data for analysis is fundamental to developing and implementing new ideas in today’s data-driven economy. Large data quantities are a significant challenge for many businesses attempting to launch an analytics initiative. They have difficulty getting used to data from various unreliable sources, but ELT can help.
What is ELT?
Data is gathered from many locations, put into a target data warehouse, and then modified in place using a modern technique known as “extract, load, and transformation” (ELT).
Unlike data integration ETL, which necessitates transforming data before loading, ELT doesn’t.
ETL & Data Integration allows the data warehouse to serve as the location for all three operations (cleaning, enrichment, and transformation). Unstructured data can be stored in these systems, and massive data transformations can be carried out.
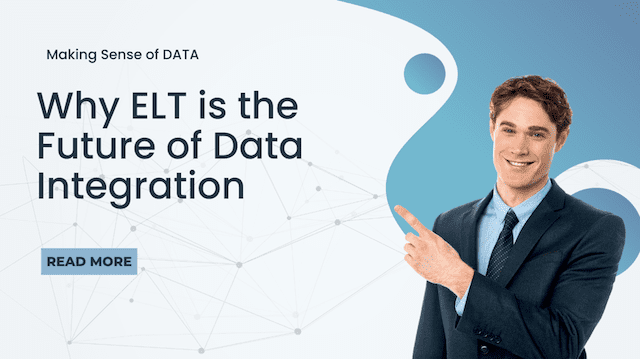
How ELT Works?
By moving the transformation process to the tail end of the data pipeline, ELT (Extract, Load, and Transformation) inverts the traditional order of the three steps. Allow me to explain the procedure.
The ETL and ELT methods of Data Management Services share the same extraction step. Data streams from virtual hardware, software, and applications are consumed in their whole or in accordance with established criteria.
ELT diverges from its archenemy ETL at the point of loading. Instead of sending the data to a temporary server for processing, ELT sends it straight to its final resting place. The time it takes to extract and transport the resource is therefore reduced.
Data is sorted and normalized in the ELT database or data warehouse, and a subset or the entire set is retained for future use in reports. The cost of holding this much information is greater, but it may be mined for timely, actionable business insights.
Here you can learn more about the case study that ETL applying wholesale & distribution data analytics solutions
Difference Between ETL and ELT
Where and how data is transformed and stored in data warehouses is the key distinction between ETL and ELT.
There are primarily two distinctions between ETL and ELT. There are two key distinctions between traditional databases and data warehouses: the location of data transformation and the method of data storage.
Organizational factors, data storage architecture, and fundamental storage technologies all play a role in deciding whether an enterprise should employ ETL or ELT for a given data management use case.
Why ELT Is the Future of Data Integration
Using ELT, companies can benefit from big data’s effectiveness and analytical power. The elimination of waste, delays, and other obstacles speeds up the process and makes it more efficient. Advantages of ELT include, but are not limited to:
Flexibility of Information
When combined with a Data Factory, ELT makes it simple to incorporate new and varied data sources into the process by ingesting data in any format, whether structured, semi-structured, or unstructured.
1. Superior Scalability
ELT can rapidly and effectively extend your cloud storage space when your data needs grow. When it comes to managing data ingestion at scale, you won’t have to reinvent the wheel as you could with ETL.
2. Speed
Since transformation occurs at the end of the ELT process, data may be loaded more quickly, and information can be accessed without delay.
3. Low Price
Since ELT can automate the process of onboarding data with little effort, it can reduce the total cost of ownership. Also, because it is hosted in the cloud, there is no need to buy any equipment.
4. Quicker Deployment
This is because the transformation takes place after the load function, after the migration has been completed, eliminating any potential delays that may have been caused.
To summarize, ELT separates the data transformation phase from the preceding extraction and loading phases. Data extraction and loading can be done independently of data transformation, eliminating the need for individualized data extraction and loading results. In light of this and the maturation of the cloud, the extraction and loading may be automated, outsourced, or scaled to meet changing demands.
Conclusion
Improvements in data quality and integrity can be achieved through ETL and ELT. The optimal course of action will rely on the many variables we’ve already covered.
Increasingly, the ELT process is being used instead of ETL. Therefore, it wouldn’t be inappropriate to name ELT the new king of data management. Sources with in-depth knowledge, including considerable data modeling and analytics expertise, are required to maximize ELT’s potential.
All things considered, a reliable software provider is exactly what you need.
Businesses in the healthcare, education, telecommunications, retail, financial services, etc. industries can benefit from Data Nectar’s cutting-edge business intelligence, data visualization, and advanced analytics solutions. These tailored BI systems analyze raw and processed data to conclude the business, taking into account the particulars of the sector and the way it functions.
As cloud infrastructure prices decrease and ELT platforms develop, more and more companies will recognize the wisdom of adopting ELT Data Integration Services.